Salesforce Einstein Delivers More Than One Billion AI-Powered Predictions Per Day
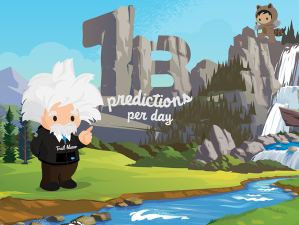
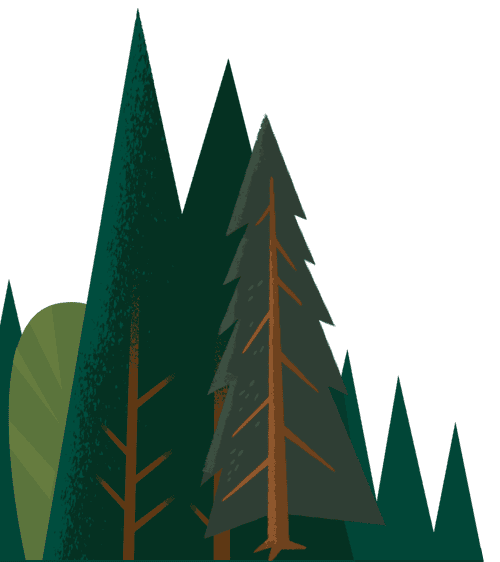
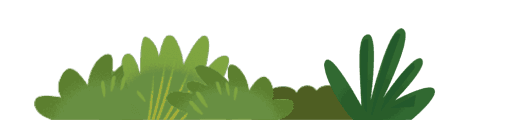
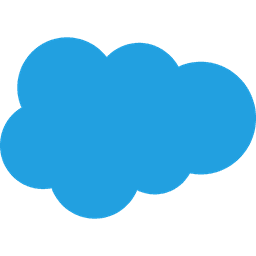
jjoseph@salesforce.com
More data is available today than at any other time in history. IDC forecasts that 163 zettabytes of data will exist by 2025 — with each zettabyte equivalent to one trillion gigabytes. All this data could unlock a new world of business opportunities. However, companies are struggling to harness their data to answer the day-to-day questions that drive their businesses forward: Which deals are most likely to close? Which customers are most likely to unsubscribe from marketing emails? Which service cases should be prioritized?
With Salesforce Einstein, every business user is armed with AI-powered technology to make sense of their data and help answer these questions– and many more– empowering them to be more productive, make smarter decisions and deliver more personalized customer experiences. Today, we are excited to announce that Einstein is now delivering more than 1 billion AI-powered predictions every day.
This incredible milestone is made possible by Einstein’s automated machine learning framework. Nicknamed Optimus Prime internally, the framework uses AI to automatically create personalized AI models and predictions for each customer, at scale. Three years ago, VP of Data Science Vitaly Gordon, Sr. Director of Data Science Shubha Nabar, and Principal Data Scientist Leah McGuire, with the help of a small team of data scientists, set out to build the framework, which leverages the extensive data that customers already trust to Salesforce’s multi-tenant platform.
In the following Q&A Vitaly explores the technology that fuels Einstein and how the team is building custom AI for Salesforce customers.
What were the unique challenges your team faced in creating Einstein AI?
Unlike consumer AI where one app works for millions of users, there is not a one-size-fits-all solution for enterprise AI. Each business has a unique set of needs and processes, and enterprise tools must be customizable to support a vast array of complex – and in some cases unknown – business workflows. In addition, every company has different data, from different sources and in different formats that vary wildly across each department. Einstein is able to build individualized AI models at scale for every customer’s unique set of data, workflows and processes.
This meant that models had to be built, trained, and hand-tuned for each individual customer and use case. With 150,000+ customers, the only way to pull off something of this scale without hiring tens of thousands of data scientists is to use AI to automate this process, and that’s exactly what my team and I did to build Einstein. We built AI to build AI.
How does Einstein’s automated machine learning (ML) framework work?
Einstein was born out of the basement of our Palo Alto office. Shubha and I set out to build the auto-ML framework directly into Salesforce’s multi-tenant platform so that AI models are automatically built and personalized for every tenant, or customer, of an application. Everything from data cleaning, data balancing, feature generation, and feature engineering to feature selection, model selection, and score calibration is automated by Einstein.
For a good analogy, compare a medical specialist to a veterinarian. A specialist studies within the same field for many years, sometimes specializing in the workings of a very particular organ or even a part of an organ. This is how most of the AI in the world is done. People tend to specialize in a very particular type of problem and solve it in a very precise manner. On the other hand, a veterinarian works with a wide variety of animals, from horses to cats. While no veterinarian has ever operated on every single animal, he or she will still know how to make logical deductions for an animal that they’ve never seen. This is what we are trying to do with Einstein. We want to give it a general understanding of artificial intelligence so it can solve problems that it has never observed before, on datasets that it has never seen before.
The secret sauce for this level of automation is metadata. While we humans never see what the data actually is, the metadata tells us whether the field is text or a simple numeric field, a category, phone number, email address, latitude and longitude coordinates, currency, or a percentage. In addition, the metadata also connects the dots between seemingly different pieces of data in the database and identifies how they relate to each other in order to generate even more predictions. With this, Einstein’s auto-ML framework is able to augment and engineer these fields in numerous ways and generate a rich set of predictors for each individual use case in a matter of hours, instead of weeks or months.
What are some examples of the predictions that customers are building today?
Einstein’s predictions are as unique as the companies using them and come in many forms. There are predictions about how likely a sales lead is to convert to an opportunity, which customers to offer a discount to, whether a service case will escalate, which products to recommend together and estimations of forecasted account values.
A leading U.S. bank is using Sales Cloud Einstein and Einstein Discovery to predict which prospects to prioritize in the sales cycle. For example, based on financial goals, center-of-influence relationships, life events and other variables for each prospect, Salesforce Einstein provides relationship managers and loan officers with recommendations on how to best engage with them, leading to higher levels of conversion, customer satisfaction and retention.
Another great example is a modern furniture brand Room & Board, which is using Marketing Cloud Einstein to better align its online customer experience with its in-store experience by optimizing product recommendations in every email. As a result, the company has seen a 150 percent higher conversion rate for customers who engage with predictive content and then click to buy. In addition to customer experience improvements, staff productivity has increased because employees are no longer responsible for manual creation of promotions and offers.
A lot has happened in the three years since you started building Einstein. What has surprised you most along the way?
When we started building Einstein the scale and variety of the ML problems we had to solve for was daunting. We got to work automating AI and when we turned Einstein on for our first pilot customers we knew we were on to something. The system immediately started generating customer-specific models without any human intervention or input and with such incredible accuracy and speed that we thought there was an error in our monitoring dashboards. I don’t know how Alan Turing felt when his machine cracked the first Enigma code, which of course was far more significant and impacted the course of history, but I like to imagine that our feelings of excitement were similar.
Seeing this kind of momentum is what has motivated us from day one but we’re still truly at the tip of the iceberg. There are so many more predictions to make and use cases to serve and I’m excited to see where Einstein takes us next.
Congratulations to the entire team that helped make these one billion predictions a reality. Now onto the next billion!
Editor’s Note: Salesforce’s Einstein team is seen in the photo below: