How to Get Started With AI: 4 Steps to Define Your Business Use Case

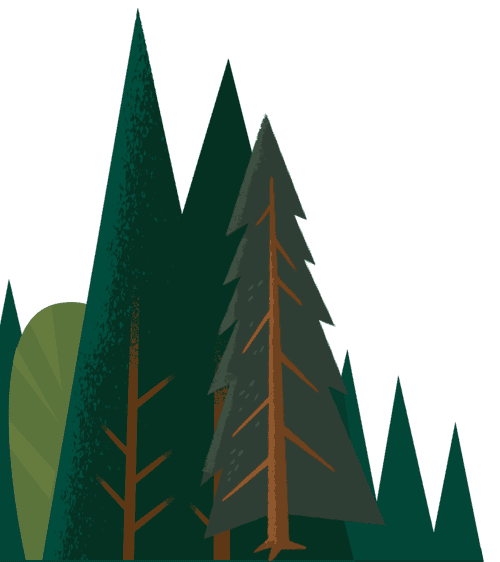

Wondering where to start with enterprise AI? Check out this video and article for 4 steps to define your AI use case.

Marco Casalaina
A recent Google search for the question, “What can AI do?” returned 2.7 billion results. The sheer volume of web pages that speak to AI’s capabilities, real-world applications, and separate hype from reality demonstrate two things:
First, people are interested. Second, they’re confused.
It’s no wonder. We hear pundits, pontificators, prognosticators (and, yes, some tech companies) talk about AI as the magic elixir that cures all ills. It’s the business game-changer that you need to have — now.
But how do you know if your business would actually benefit from AI? What specific business areas would benefit most between sales, marketing, customer service, and more?
And then you have to consider what AI can do. What can’t it do? What are the use cases? Who needs to be reskilled?
The questions around AI are as endless as its potential. This has created a disconnect between interest and execution. Need proof? According to a study by MIT Sloan Management Review and Boston Consulting Group, 84% of executives believe AI will create a competitive business advantage. What’s more, our research has found that 62% of your customers are open to you using AI to improve the experience you give them. And yet, fewer than half — according to MIT just 38% — have actually implemented an AI strategy.
This is the first article in our new “Ask Salesforce” series, explaining how to use AI in your business. We’ll start with how AI can help you make better decisions and answer key business questions.
 
1. Start with a concrete question
Step one in making AI work for your business is to get clear on the specific question you want AI to answer.
Can that question be represented as a yes-or-no question, like “Is this customer going to churn or not?” Is it a numerical question, like “How much revenue will this customer bring in?” Or is it a matching question, like “What is the best product to recommend to this customer?”
The yes-or-no question
Yes-or-no questions are some of the most common questions that AI can answer. Something like “Is John Smith a good lead for my business?” seeks to answer the question of whether a prospect will become a customer and the answer is binary — yes or no. When you apply AI to this question, it will learn from the historical data you’ve stored in your systems, and will then be able to produce predictions based on what it’s learned.
Every AI initiative needs to start with what you’d like to know, what you’d like to achieve, and what you’d like to predict.
Continuing the example, the prediction for whether John Smith will become a customer will generally come in the form of a probability: “John Smith has a 35% chance of becoming a customer.”
These probabilities are sometimes converted into scores. Instead of showing the raw probability, one might instead assign a “100” to the lead with the highest probability of conversion and a “0” to the lead with the lowest probability, with all the leads in between getting scaled accordingly.
The numerical question
Some business issues do not fit neatly into the yes-or-no question paradigm. Fortunately, you can also phrase questions in numeric terms. For example, “How much new revenue will John Smith bring in?”
Once it’s learned from your data, AI will respond to this type of a question in the form of a number, like “John Smith is predicted to generate $40,000 of revenue over his lifetime as a customer of ours.”
The matching question
If your business issue does not fit the yes-or-no or numeric question paradigms, never fear. There is yet a third form of question you can ask of AI: the matching question. The most common form of this is found in ecommerce: “What is the best product to recommend to this customer?”
However, there are numerous other variants of this question. In a recruiting operation, it might be “What is the best job posting for this candidate?” or the converse, “What is the best candidate for this job posting?” In a marketing context, it might be “What is the best email to send to this recipient?”
The point is, every AI initiative needs to start with what you’d like to know, what you’d like to achieve, and what you’d like to predict. Once you’ve fixed upon a concrete question on which you’d like your AI to focus, you can then determine whether or not you have sufficient data to answer that question.
2. Make sure your data answers the question
A simple rule of thumb for AI is if you can’t report on a question historically, you can’t predict it. You need to be able to build a report that collects all the data you have that answers your question up until now, so AI can use it to predict answers for the future.
For example, if your question is “Will this customer churn or not?” you need to be able to build a report for all of your historical customers that shows exactly which customers have churned and which have not. In the case of churn, sometimes this is simple — if you have a subscription business, a customer who has unsubscribed has clearly churned.
If you can’t report on a question historically, you can’t predict it.
However in other businesses, the definition of “churn” may be more nebulous, like “a customer has churned if she’s not purchased anything from the business in the last six months.” In the latter case, you may need to transform your data to some degree to ensure that it answers your question historically.
Businesses need to answer the following key questions to assess AI readiness:
- Is your data in a place where AI see it?
- Is the data aligned with your use case?
- Is the data you plan to feed to your AI free of unwanted bias?
- Is your data reasonably clean?
The success of your AI program has its foundation in the quality, volume, and categorization of data. You must have the historical data required for the AI algorithms to find and extract patterns for predictions.
As MIT noted in its report, “No amount of algorithmic sophistication will overcome a lack of data.”
3. Determine how to use your AI prediction in your business
Once you have a clear question and the data to answer it, you’ve built your AI prediction engine.
Your next challenge is deciding on the best way to share that information with the people who can use it. In some cases, it’s as simple as putting that number in front of the right employees. To continue our previous example of churn prediction, this might mean that your customer record prominently shows a new number to indicate a customer’s predicted likelihood of churn.
Some predictions are better displayed en masse — if you have 2 million customers of which 50 thousand have a high likelihood of churn, it may not be feasible for people to look at every individual customer record, and so the predictions may be better exposed as a list.
Ultimately, an AI prediction is just a number. It still requires a human to take action on it.
Sometimes you may not choose to expose the predictions themselves at all, but instead, show recommendations derived from those predictions. Perhaps instead of telling your users which customers are likely to churn, a better strategy is to incorporate the predictions in a rules workflow or next-best-action system and use those systems to produce recommendations. For example, “if a customer has more than 30% likelihood of churning, go visit them; if it’s between 20% and 30%, call them; etc.”
Ultimately, an AI prediction is just a number. It still requires a human to take action on it. The goal is to present that prediction to the people that can use it in a manner that best allows them to turn that prediction into action.
4. Consider how AI will impact workflows and responsibilities
AI represents a sea change not only in how you make decisions that drive your business but also in how your business itself is run. Roles and responsibilities across your entire organization will change.
As a result, you must consider:
- Whether the right stakeholders are involved in your efforts
- How existing workflows will be impacted
- How you will demonstrate ROI for your defined AI use cases
For instance, just knowing that a customer has a 25% likelihood to churn is not enough. You need to take action on that information (like running a retention campaign) and new workflows and rules will give you that power.
Business leaders need to encourage information sharing and set the tone by consolidating fragmented data from across different departments
Finally, consider and mitigate any “turf wars” that may emerge around data ownership. In these cases, business leaders need to encourage information sharing and set the tone by consolidating fragmented data from across different departments.
Otherwise, training for AI systems will be incomplete and any business value, while still possible, will be made that much more difficult to achieve.
We know that AI will continue to have a huge impact on our lives and work. It sometimes seems a daunting change to manage, but there are tools and examples out there to help you succeed. Getting started with enterprise AI is all about the right questions, the right data, and the right level of transparency with employees and customers.
If you want to learn more, visit Trailhead for free learning modules on specific applications of AI that can work for your business.